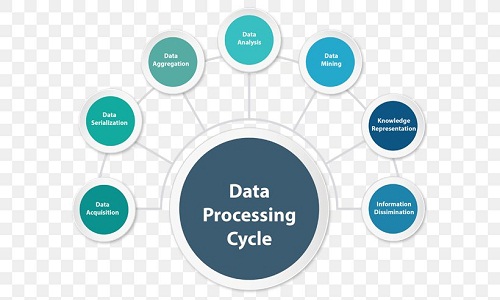
Data processing is the practice of refining the collected and unstructured datasets. The motive is to clean and structure them for using or integrating with the business intelligence.
But, it’s not easy. This practice needs data scientists, solution architects, and data experts to work together. However, automation software is easily available to reduce the burden of manual processing.
Some smart data mining companies or processing units smartly integrate manual workforce and applications together to produce benchmark quality. The entire process covers the collection, cleansing (filtering and sorting), processing, analysis, and storage of data from niche-based resources.
With this happening, various organizations find ways to introduce convenience, fast turnaround, enhanced security, and more productivity. These all happenings positively impact the pan business.
Type of Data that Can be Processed
Not every detail can be processed. However, everything that you read, write, and share is a piece of data. For many of us, data mean any number of statistics. But actually, it can be any images, graphs, survey answers, transactions, and a lot more things.
In short, data are broad and can be categorized into personal information, financial transactions, banking details, etc. Depending on what type of details you want to process, experts define the process accordingly.
Stages of Data Processing
Mainly, there are six stages that are commonly followed by big data entry or mining companies.
Data Collection
This is the first step wherein data are pulled from various sources, which can be the cloud storage, CRMs, websites, or any disparate systems. Many companies hire inexpensive options like data entry from India or an Asian country to save money & get quality. They can also provide data from data lakes and warehouses. The purpose is to translate crude details into insights so that the decisions can be drawn easily. For example, customer behavior and their interest can be discovered via website cookies to display niche-based ads.
Data Preparation
Upon data collection, these sets are prepared. For this purpose, they are all organized, cleansed thoroughly, and verified. All typos, errors, inconsistencies, spaces, blanks, and incomplete details are fulfilled. Being a time-consuming practice, software like SolveXia can be deployed to do it in a few minutes.
Input Details
Here, the datasets are prepared to communicate with the system. This system can be a CRM or data warehouse. Herein, the collected sets are transformed into a language that the machine can understand. Thereafter, the information starts taking a structural shape.
Data Processing
This phase is dedicated to processing via algorithm and machine learning models. The data scientists and programmers write codes to specify how particular processing will go on. For instance, a PDF conversion can be done with the codes or scripting that runs to automate scanning, storing, processing, and then, cleansing the file.
Output
Once processed, the result is turned into visual data. At this point, the understandability is focused. So, the transformed datasets are mold into graphs, plain text, images, charts, videos, etc. This practice makes information comprehensive for everyone.
Define Storage
Finally, the processed files are turned ready to draw benefits. The experts and analysts leverage insights to define how they can be useful for administering business problems. But, this is not the end of usage. It may be used further. This is where data storage comes out in handy.
Besides, robust storage is a necessity for being compliant with policies like GDPR, which ensures the security and confidentiality of personally identifiable information.
Practices that Can Be Used in the Future
This is the fact that businesses access information every second from a variety of sources. But, the big challenge here is decentralized datasets. In the future, the information would be centralized.
Now, the concern is where.
Certainly, we have cloud technology. It is where data can be processed at the point of entry and hence, the exact information can be collected in a fast turnaround time. Also, this platform offers robust security by preventing hackers from getting in.
Additionally, cloud platforms are affordable. They are extremely cost-effective than having to store data on servers on-premise. This is the most compatible technology, which lets you work from home or anywhere. The data remain accessible to authorized people from remote regions.
Types of Data Processing
Here is the roundup of what type of data can be processed.
Batch Processing
It is ideal for large amounts of data. The systems like Payroll can be managed effectively through this batch processing.
Online Processing
Online processing allows continuous processing. It means that you can enter and use data online. For instance, entering a barcode via a scanner for recording transactions can better explain online processing.
Real-time Processing
This is applicable to small-scale databases. For instance, the machines like ATMs produce real-time data, or Inventory Management systems create data on Google Spread Sheets for quick analysis and monitoring.
Time-sharing
To let several users use the same computer resources, data can be processed in time slots.
Multiprocessing
It splits data into frames and processes using more than one CPU in a system. Weather forecasting is its finest example.
Practices of Data Processing
These can also be called the methods of data processing. There are certain ways to refine and transform any file or records into understandable format, which is called processing.
Here are some common practices that are followed for processing:
1. Manual Data Processing
As the name suggests, manual data processing is a tedious job. It is performed by hand without using any machine, or software. People visit or interact with end-users to gather information. Then, the paper-bound processing, which includes collection, compiling, filing, cleansing, calculation, analysis, and standardizing of collected samples.
Despite being inexpensive, this method may prove costly. It is simply the erroneous datasets that may strain the marketing budget because of errors.
2. Mechanical Data Processing
This method involves tools and devices, like calculators, scanners, printers, etc. These devices are fast and would not strain your budget due to errors in the datasets. Besides, this processing does not involve any human playing a massive role.
3. Electronic Automated Data Processing
This is the most efficient, and cost-effective practice, wherein errors are minimum. The end-to-end processing is carried out automatically using programs and software.
However, it involves upfront investment in applications and experts. But, these all are compensated when you get completely clean and useful insights that have limitless opportunities for business growth and maximizing revenues.
Summary
The advent of automation technology has reshaped how businesses can use data and their intelligence. Electronic data processing is the best practice that let you proactively process, and manage the accuracy of the whole database. This is the best practice of processing files or records.
Be the first to comment